MDW Software Usage - Beginner's Guide & Tips
What does this specific methodology contribute to a given field? A deep understanding of a particular methodological approach is essential for its effective application.
The phrase "methodological approach" describes a structured system of techniques and procedures used in a specific area of study. It often includes a set of principles, assumptions, and tools designed to achieve a desired outcome or result. A robust methodological approach can yield reliable and valid findings, enabling researchers to draw meaningful conclusions. For example, within the field of scientific research, a detailed methodology ensures consistency and allows others to replicate the study, thus contributing to the validity and generalizability of the findings.
A well-defined methodology fosters consistency, reproducibility, and ultimately, enhances the rigor and credibility of research. Its structured approach provides a framework for systematic data collection, analysis, and interpretation. Consequently, this strengthens the basis for theory building, problem-solving, and the expansion of knowledge in various disciplines. This approach, whether applied in science, social sciences, or any other field, contributes significantly to the cumulative advancement of knowledge and understanding.
Moving forward, let's explore various methodologies used in specific contexts. By examining diverse methodological approaches, we can analyze their strengths and limitations, understand their contexts of use, and appreciate their value in different domains of inquiry.
Uso MDW
Understanding the key aspects of "Uso MDW" is crucial for effective application. This involves a multifaceted approach, encompassing practical application, theoretical underpinnings, and relevant contexts.
- Data analysis
- Model development
- Workflow design
- Validation procedures
- Interpretation
- Implementation
- Evaluation metrics
- Ethical considerations
These aspects, individually and collectively, represent the fundamental components of a robust methodology. For example, data analysis forms the bedrock of model development, while rigorous validation procedures ensure the reliability of results. Effective implementation requires careful workflow design and selection of appropriate evaluation metrics. Finally, awareness of ethical considerations is crucial for responsible application of the methodology. Understanding these interwoven aspects is essential for a comprehensive grasp of "Uso MDW," whether in academic research or practical application.
- Dan Amp Shay Net Worth 2023 A Deep Dive Into Their Success
- Adorable Dana Dolls Exquisite Collectibles More
1. Data analysis
Data analysis is a fundamental component of "uso mdw," playing a crucial role in understanding and interpreting information. Effective data analysis provides a foundation for model development, validation, and ultimately, informed decision-making. This process involves a systematic examination of data, utilizing various techniques to extract meaningful insights. The results inform the development and refinement of models, ensuring a more effective and targeted approach. Examples include the analysis of market trends to predict future sales, or the evaluation of patient outcomes to optimize treatment strategies. Without rigorous data analysis, "uso mdw" lacks a crucial element of objectivity and can lead to inaccurate conclusions.
The importance of data analysis within "uso mdw" stems from its ability to identify patterns, trends, and correlations within complex datasets. This allows for a more nuanced understanding of the subject matter, facilitating the creation of more accurate models and the development of effective strategies. In practical applications, such as financial modeling, the analysis of historical data is crucial for forecasting future market performance and managing risk. Similarly, in scientific research, data analysis guides the formulation of hypotheses, facilitates experimentation design, and confirms or refutes existing theories. This process is essential to any robust methodology and significantly impacts the validity and reliability of conclusions drawn.
In conclusion, data analysis is intrinsically linked to "uso mdw." Accurate and comprehensive analysis is essential for the effective application and refinement of any given methodology. The insights gained from data analysis drive model development, enhance strategic decision-making, and ultimately, contribute to a more robust and reliable understanding of the subject matter. This, in turn, significantly impacts the quality and validity of any conclusions drawn.
2. Model Development
Model development is a critical component of "uso mdw," representing the construction and refinement of representations of complex systems or phenomena. Effective models are essential for predicting outcomes, understanding relationships, and making informed decisions. This process relies on the rigorous analysis of data, enabling the creation of tools for simulation, optimization, and prediction.
- Data Input and Selection
The quality of a model hinges on the quality and relevance of the data used in its construction. Carefully selecting and preprocessing data ensures accuracy and reliability. In financial modeling, historical market data, economic indicators, and company performance metrics are crucial inputs. Failure to account for biases or inaccuracies in input data will compromise the validity of the resulting model, leading to misleading predictions or incorrect conclusions about the phenomena under investigation.
- Model Structure and Assumptions
Model development entails choosing a suitable structure (e.g., linear, non-linear, probabilistic) that appropriately captures the relationships between variables. Explicitly defining the assumptions inherent in the model is crucial for transparency and understanding its limitations. In climate modeling, assumptions about atmospheric processes, greenhouse gas emissions, and feedback loops significantly affect the projected outcomes.
- Validation and Refinement
Validation processes are essential for evaluating a model's accuracy and predictive power. Testing the model against existing data or independent datasets helps identify weaknesses and areas for improvement. Refinement through iterative adjustments to the model's structure, parameters, or assumptions enhances its predictive capabilities and reduces errors. In epidemiological modeling, validation against historical disease outbreaks helps refine the model's accuracy and forecast future trends.
- Implementation and Application
The practical application of a developed model is often crucial. Successful deployment requires careful consideration of implementation strategies, workflows, and potential limitations. Integration of the model into existing systems and workflows is vital for realizing its intended benefits. In supply chain management, models predicting demand fluctuations inform inventory control and resource allocation strategies, leading to optimized operations.
The various facets of model development contribute significantly to the overall "uso mdw" methodology. The quality and accuracy of models directly impact the reliability of insights derived. Comprehensive model development, encompassing data selection, structural choices, validation procedures, and effective implementation, ensures the robustness and usefulness of the methodology in diverse application areas.
3. Workflow Design
Workflow design plays a critical role within "uso mdw" as it establishes the structured sequence of tasks and processes required for effective implementation and execution. A well-defined workflow ensures consistent application of the methodology across different phases, from data collection to interpretation. A poorly designed workflow can introduce inefficiencies, inaccuracies, and inconsistencies, potentially leading to compromised outcomes. Effective workflow design is, therefore, essential for the reliable and reproducible application of "uso mdw." In essence, workflow design acts as the operational blueprint for "uso mdw," guiding actions and ensuring a systematic approach to achieving the desired outcomes.
Practical examples illustrate the importance of workflow design. Consider a research project using "uso mdw." A well-designed workflow might specify precise steps for data collection, model development, validation, and interpretation. This clear structure ensures data consistency, facilitates reproducibility, and minimizes potential errors. A similar concept applies in a business context; a streamlined workflow for product development or customer service operations can significantly improve efficiency and reduce costs. Efficient workflow design in these contexts minimizes redundancies, streamlines processes, and empowers teams to operate more effectively, thereby contributing directly to the intended goals. The specific design elements vary depending on the context and complexity of the methodology but generally center on clarity, efficiency, and minimizing error.
In conclusion, workflow design is not merely a supplementary element but an integral component of "uso mdw." A well-structured workflow supports the consistent and reliable application of the methodology, contributing significantly to the quality and validity of results. Effective workflow design is pivotal for minimizing errors, maximizing efficiency, and ensuring reproducibility. By clearly outlining tasks and processes, workflow design provides the framework for a systematic and accurate approach to applying "uso mdw," ultimately impacting the efficacy and reliability of the outcomes. Any methodological approach reliant on consistent execution requires a robust workflow design to support it.
4. Validation Procedures
Validation procedures are indispensable components of any robust methodology, including "uso mdw." Their purpose is to assess the accuracy, reliability, and validity of the methodology's outputs. This crucial step ensures the findings derived from "uso mdw" are not based on flawed or unreliable processes. Without rigorous validation, the results lack credibility and undermine the very foundation of any claims made. The efficacy of "uso mdw" depends directly on the thoroughness and rigor of its validation procedures.
The importance of validation procedures extends beyond mere verification. They identify potential weaknesses and limitations within "uso mdw" itself, allowing for refinements and improvements in future applications. Consider, for example, a financial model used to predict market trends. Validation procedures might involve comparing model predictions to historical data and independent market analyses. If the model consistently deviates from reality, it highlights areas needing adjustment within the "uso mdw" framework. Similarly, in scientific research, validation procedures might include replicating experiments and comparing results to established theories. Discrepancies pinpoint necessary modifications to ensure reliable and accurate results. In essence, validation procedures are crucial for maintaining the integrity and reliability of "uso mdw" in any context.
In conclusion, validation procedures are not an optional add-on but an integral part of "uso mdw." Their role goes beyond simply confirming the accuracy of results. They identify potential flaws, provide feedback for methodological improvements, and establish the credibility and reliability of the entire process. Failing to incorporate robust validation procedures in "uso mdw" undermines the methodology's value and validity, leading to potentially inaccurate interpretations and questionable conclusions.
5. Interpretation
Interpretation within "uso mdw" represents the crucial process of extracting meaning and insight from the results generated by the methodology. It's not merely a summary of findings; rather, it's a critical analysis of data, models, and workflows, aiming to understand the implications and applications of the "uso mdw" output. This phase demands careful consideration of context, potential biases, and the broader implications of the findings. The quality of interpretation directly impacts the value and usefulness of the "uso mdw" process.
- Contextual Understanding
Interpretation requires a deep understanding of the context within which "uso mdw" is applied. Factors such as historical trends, industry specifics, or the particular characteristics of a dataset all influence the meaning extracted from the results. Interpreting market research data, for example, necessitates understanding the current economic climate and competitive landscape. Failure to acknowledge contextual elements can lead to misinterpretations and inaccurate conclusions. A thorough understanding of the context within which "uso mdw" is applied is essential for accurate interpretation.
- Bias Recognition and Mitigation
Subjectivity and bias can influence interpretation. Recognizing potential biases in data collection, model assumptions, or even in the interpreter's own perspectives is crucial. For instance, a study on social media trends might reflect biases in the data collection methodology if certain demographics are disproportionately represented. Addressing and mitigating such biases through careful scrutiny and employing appropriate controls is vital for producing reliable and objective interpretations.
- Communication and Dissemination
Effective interpretation involves clear and concise communication of findings. This includes crafting narratives that effectively convey insights to diverse audiences, from technical experts to lay readers. For example, researchers might present model predictions in a manner accessible to stakeholders, highlighting key implications and recommendations for action. This requires a balance between technical detail and understandable language, fostering a clear understanding of the implications for the intended audience.
- Critical Evaluation of Limitations
Understanding the limitations of "uso mdw" and its results is essential for accurate interpretation. Acknowledging any inherent biases or limitations of the data, models, or the methodology itself is paramount. For instance, a model's predictive power may be limited by external factors beyond its scope. This rigorous evaluation helps provide a nuanced and balanced perspective on the implications of the findings, allowing for a realistic assessment of their usefulness and relevance.
In conclusion, interpretation is not a passive process but an active engagement with the outputs of "uso mdw." By carefully considering the context, recognizing and mitigating biases, communicating findings clearly, and critically evaluating limitations, the process enhances the practical value and reliability of the entire methodology. This ensures that the insights derived from "uso mdw" contribute meaningful understanding and actionable outcomes.
6. Implementation
Implementation, within the context of "uso mdw," signifies the practical application of the methodology's principles and procedures. This phase bridges the gap between theoretical development and tangible results. Effective implementation ensures the methodology translates from abstract concepts into concrete actions and measurable outcomes. Without successful implementation, the value of "uso mdw" remains theoretical, failing to achieve its intended purpose. A crucial aspect of implementation is aligning the methodology with existing systems, processes, and resources. The success of this integration determines the efficiency and effectiveness of applying "uso mdw."
Real-world examples highlight the significance of implementation. Consider a financial institution utilizing "uso mdw" to enhance risk management. Successful implementation involves integrating the methodology into existing trading platforms and risk assessment models. This necessitates adapting workflows and training personnel on the new procedures. Failure to effectively implement the methodology would result in a gap between theoretical risk mitigation strategies and operational reality. Alternatively, in scientific research, implementing a new methodology for data analysis involves training researchers, acquiring necessary software, and adjusting existing experimental protocols. Successful implementation leads to consistent and accurate data analysis, contributing to reliable and impactful findings. The outcome hinges on how well the methodological framework integrates with existing scientific infrastructure. Successful implementation hinges on factors such as resource allocation, personnel training, and adaptable workflows.
In summary, implementation is not a passive step but a critical component of "uso mdw." It validates the methodology's potential and determines its practical utility. Challenges during implementation often stem from misalignment between the methodology and existing systems or a lack of adequate resources and training. Addressing these issues, by carefully considering and adapting the methodology to the specific context, ensures a smooth transition from conceptual framework to practical outcomes. A thorough understanding of implementation, within the framework of "uso mdw," is crucial for maximizing the methodology's impact and achieving its intended goals.
7. Evaluation Metrics
Evaluation metrics are integral to "uso mdw" because they provide a structured approach to assessing the success and effectiveness of the methodology's application. These metrics quantify the results, enabling objective comparisons and informed decisions about the methodology's strengths, weaknesses, and areas for improvement. Without appropriate evaluation metrics, it is difficult to ascertain whether "uso mdw" yields meaningful outcomes. The selection and application of metrics are critical for drawing accurate conclusions about the methodology's overall impact.
The specific metrics used will vary depending on the context of "uso mdw." For instance, in a clinical trial, metrics might include patient recovery rates, side effects, and improvements in health markers. In a marketing campaign, key metrics might encompass conversion rates, customer acquisition costs, and return on investment. Similarly, in a scientific study, metrics might involve precision, recall, and F1-score to evaluate model performance. Careful consideration must be given to the relevance and appropriateness of metrics in relation to the specific goals and objectives of "uso mdw." Metrics must be reliable, valid, and aligned with the target outcomes. The choice of metrics significantly impacts the interpretation of results, highlighting the importance of meticulous selection. A well-defined set of metrics fosters a clear understanding of the methodology's success and its potential areas for enhancement. For instance, a model predicting customer churn might utilize metrics such as the accuracy rate, precision, and recall to gauge the model's performance. These quantified assessments directly inform improvements to the model or subsequent application of "uso mdw." Without these metrics, there's no objective way to measure the impact or validate the usefulness of the methodology.
In conclusion, evaluation metrics are essential components of "uso mdw." They provide a standardized framework for assessing the methodology's effectiveness and impact. The careful selection and application of appropriate metrics enhance the reliability of results, allowing for informed decisions regarding modifications or adaptations to "uso mdw." This data-driven approach promotes consistent and measurable improvement in any context where "uso mdw" is employed, guiding future applications and maximizing its potential benefits.
8. Ethical Considerations
Ethical considerations are paramount when applying any methodology, including "uso mdw." The potential for misuse, unintended consequences, and the impact on individuals and society necessitate a proactive approach to ethical implications. This section explores key facets of ethical concern, underscoring the importance of responsible implementation of "uso mdw."
- Data Privacy and Security
Protecting sensitive data is paramount. "Uso mdw" frequently involves handling personal or confidential information. Robust data encryption, anonymization techniques, and adherence to relevant privacy regulations (e.g., GDPR) are critical. Examples include ensuring patient data in medical research or financial records in financial modeling are handled securely. Failure to safeguard this data could lead to significant harm, including identity theft or reputational damage. This facet emphasizes the need for meticulous data handling procedures.
- Bias and Fairness in Algorithm Design
Algorithms underpinning "uso mdw" can perpetuate or amplify existing societal biases if not carefully designed and monitored. Algorithms trained on biased data can yield discriminatory outcomes. Examples include AI systems in loan applications or hiring processes exhibiting bias against certain demographic groups. The potential for unfair or discriminatory outcomes in applications of "uso mdw" necessitates rigorous bias detection and mitigation strategies in model development and deployment.
- Transparency and Explainability
Understanding how "uso mdw" arrives at its conclusions is crucial for trust and accountability. Complex models can be "black boxes," obscuring the decision-making processes. Explainable AI (XAI) techniques and transparent methodologies are necessary for users to comprehend the logic and rationale behind model predictions. Examples of applications requiring transparency include predictive policing models and criminal justice risk assessments. Opaque decision-making can erode public trust and lead to inappropriate or unjust applications.
- Accountability and Responsibility
Determining who is accountable for the outcomes of "uso mdw" applications is essential. Clear lines of responsibility for model development, implementation, and interpretation must be defined. This includes considerations for unintended consequences, such as unexpected outcomes or biases in model predictions. Examples include the liability in automated driving systems or the accountability for financial models causing unforeseen losses. Establishing accountability mechanisms is critical to mitigating potential harms stemming from applications of "uso mdw." In cases of misapplication or errors, clear protocols for remediation and accountability should be defined.
Integrating ethical considerations into every stage of "uso mdw" from data collection and model building to interpretation and deployment is crucial. Failure to address these facets risks compromising the methodology's reliability, fairness, and societal acceptance. A robust framework for ethical considerations ensures responsible development, implementation, and use of "uso mdw" to generate beneficial and trustworthy results.
Frequently Asked Questions about "Uso MDW"
This section addresses common inquiries regarding the "Uso MDW" methodology. Clear and concise answers are provided to facilitate a comprehensive understanding of the approach.
Question 1: What is "Uso MDW," and what are its key components?
"Uso MDW" refers to a structured methodological approach, often applied in research and practical contexts. Key components encompass data analysis, model development, workflow design, validation procedures, interpretation of results, implementation, evaluation metrics, and ethical considerations.
Question 2: How does "Uso MDW" differ from other methodologies?
The unique aspects of "Uso MDW" are the integration of data-driven analysis, iterative model refinement, robust validation protocols, and a strong focus on ethical considerations. While sharing common elements with other methodologies, "Uso MDW" distinguishes itself through its emphasis on practical implementation and assessment.
Question 3: What are the essential steps in applying "Uso MDW"?
A typical application involves: data collection and analysis; model construction and validation; workflow design; implementation and execution of the process; rigorous evaluation using appropriate metrics; and, finally, ethical review and transparent interpretation of outcomes.
Question 4: What is the importance of validation procedures in "Uso MDW"?
Validation procedures are crucial for ensuring the accuracy, reliability, and validity of "Uso MDW" outputs. They help identify flaws in the methodology itself, enabling refinements and improvements for future applications. Thorough validation is essential to establish trust in the outcomes and their potential impact.
Question 5: How can ethical considerations be integrated into the "Uso MDW" methodology?
Ethical considerations are not peripheral; they are interwoven with every stage of the process. This includes safeguarding data privacy, recognizing and mitigating potential biases in algorithms and data, promoting transparency, and defining clear lines of accountability. This ensures responsible development, application, and evaluation of the methodology.
Understanding these key aspects provides a foundation for the effective application and evaluation of "Uso MDW."
Moving forward, let's delve into specific applications of "Uso MDW" in diverse fields.
Conclusion
This exploration of "Uso MDW" has illuminated the multifaceted nature of this methodological approach. The analysis has underscored the crucial role of data analysis, model development, workflow design, and validation procedures in ensuring robust outcomes. Key components, including the careful selection of evaluation metrics and a thorough consideration of ethical implications, have been shown to contribute significantly to the reliability and validity of the process. The discussion has emphasized the importance of implementation in translating the methodology's principles into practical applications and the critical role of interpretation in extracting meaningful insights from results. Furthermore, the analysis highlighted the imperative of acknowledging and mitigating biases and ensuring the transparent and responsible application of "Uso MDW." Each aspectfrom data handling to model validation to ethical considerationsis not a mere adjunct but an integral part of a cohesive methodological framework.
The "Uso MDW" methodology, when applied meticulously and responsibly, offers a powerful framework for tackling complex problems across diverse fields. However, the ultimate success hinges on a commitment to rigor and a proactive approach to ethical considerations. Continuous refinement and adaptation are essential to maintain the methodology's relevance and effectiveness in an evolving landscape. Future research and application should focus on exploring new avenues of application, evaluating the long-term impacts of "Uso MDW," and fostering ongoing dialogue on responsible methodology to advance knowledge and improve decision-making in various domains. The successful implementation and advancement of "Uso MDW" require a dedication to ongoing evaluation, a critical eye toward potential biases, and a commitment to ethical conduct.
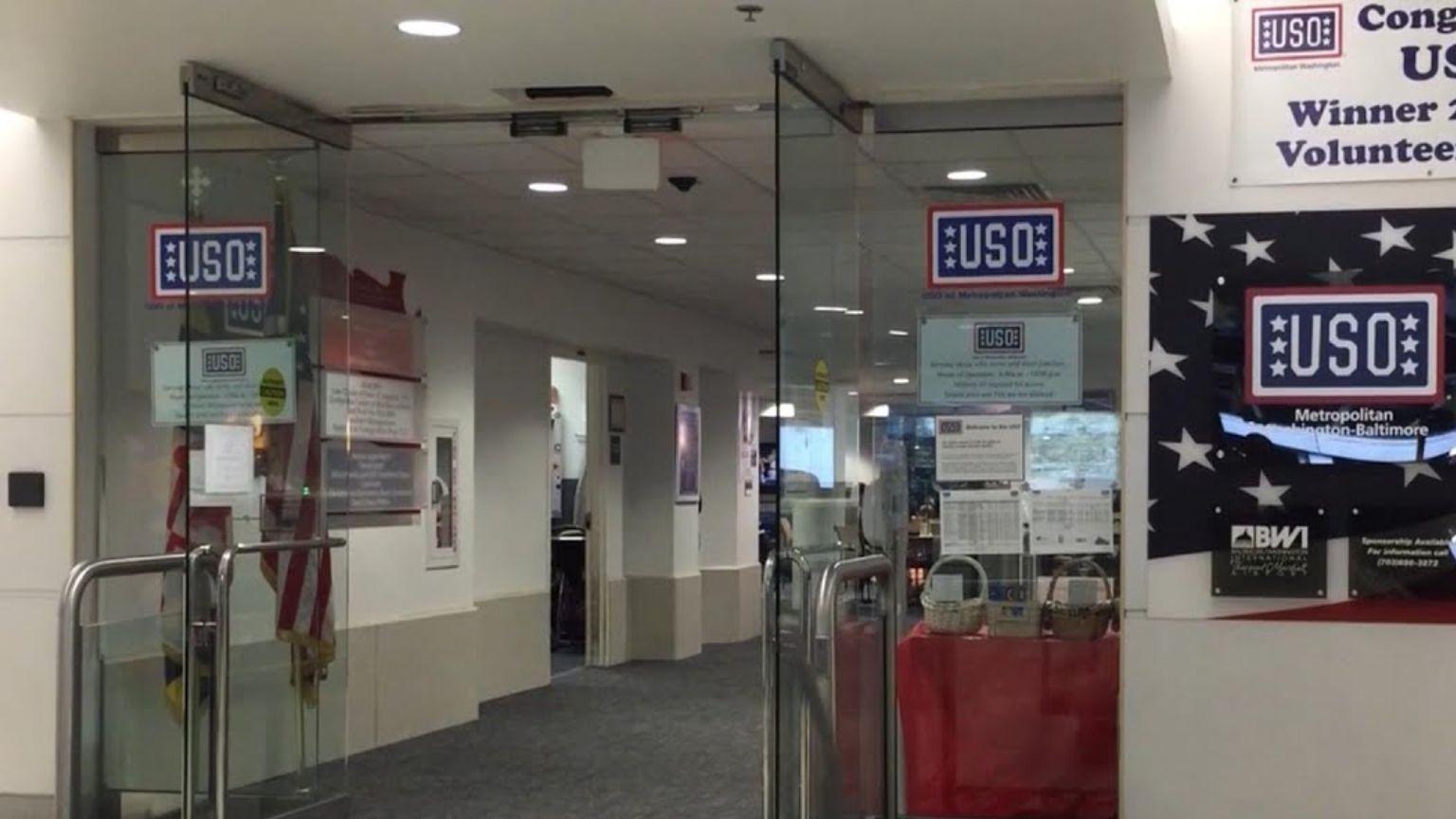

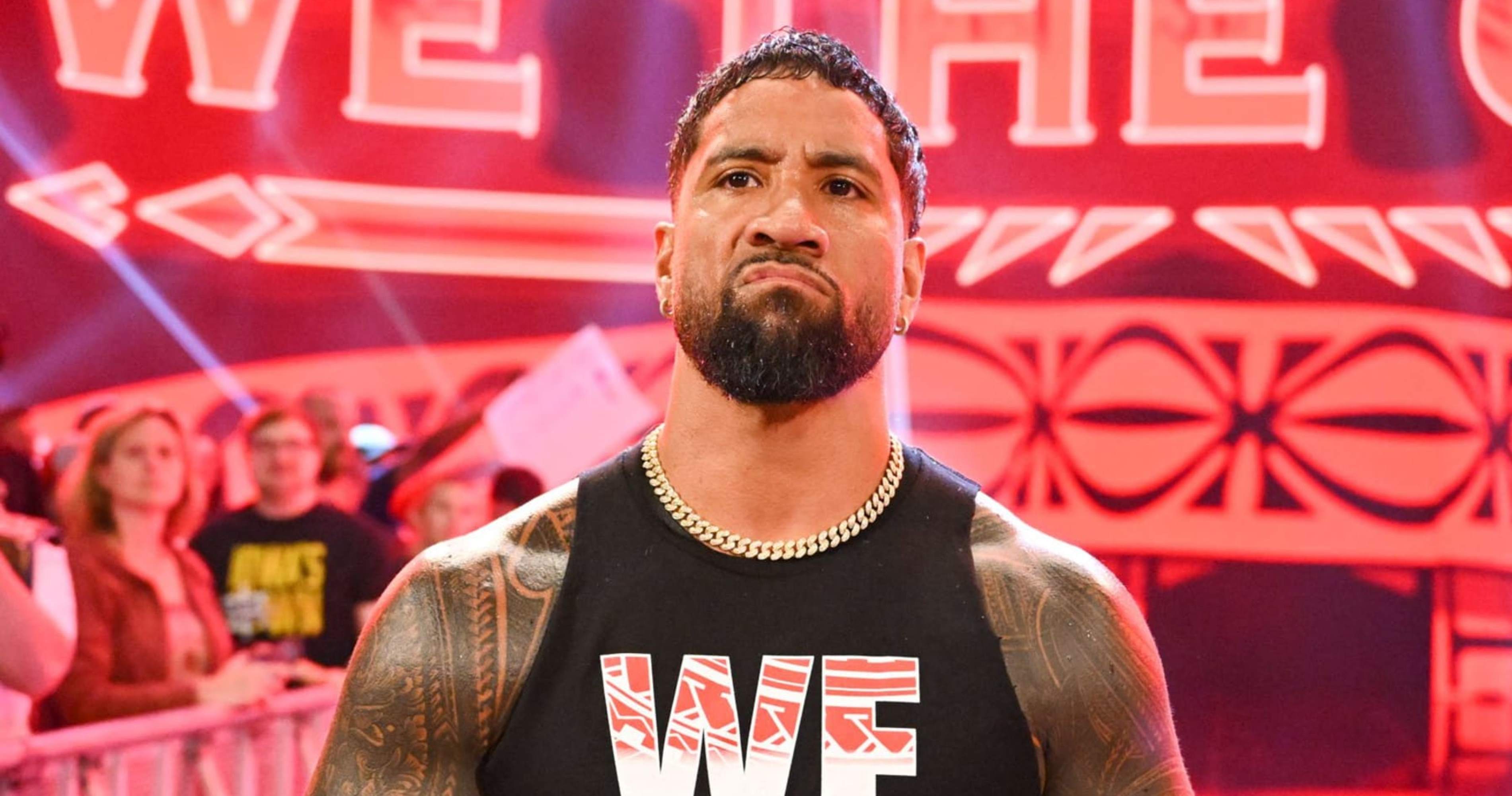
Detail Author:
- Name : Dr. Lavinia Ferry
- Username : dimitri.yundt
- Email : zkoelpin@gmail.com
- Birthdate : 2004-11-06
- Address : 86028 Anderson Brook Apt. 410 New Meaghan, NH 31212
- Phone : +17256407935
- Company : Abbott Inc
- Job : Recreational Vehicle Service Technician
- Bio : Reprehenderit provident provident corrupti minima fugit natus autem harum. Quidem assumenda doloremque doloribus odio est. Quas expedita dolorem omnis fugit provident laudantium ipsum.
Socials
instagram:
- url : https://instagram.com/rroberts
- username : rroberts
- bio : Et voluptatem optio et dolore est dolores deleniti. Voluptatem provident totam non sit.
- followers : 5027
- following : 2893
tiktok:
- url : https://tiktok.com/@ricky_roberts
- username : ricky_roberts
- bio : Mollitia soluta recusandae soluta consequatur doloremque eligendi.
- followers : 959
- following : 1659
twitter:
- url : https://twitter.com/robertsr
- username : robertsr
- bio : Doloremque quos enim quos dolores voluptas omnis perferendis officiis. Esse aut ducimus commodi aut itaque. Necessitatibus voluptas ut ullam aut.
- followers : 2378
- following : 2236
linkedin:
- url : https://linkedin.com/in/robertsr
- username : robertsr
- bio : Consequatur omnis vero velit enim rem veritatis.
- followers : 3417
- following : 2532