Phoebe Kalib's Latest Creations & Designs
What is the significance of this specialized terminology? How does it impact the field?
This term, a unique combination of words, likely refers to a specific concept, methodology, or dataset. Without further context, it's impossible to definitively define its meaning. It might represent a novel approach, a proprietary algorithm, or a unique categorization system within a particular domain. Understanding the context is crucial to grasping its implications. For example, within the realm of data analysis, this term might signify a specific type of structured data or a novel way of organizing data patterns.
The potential importance depends entirely on the field in which this term is employed. If it represents a significant innovation, it could lead to breakthroughs in data analysis, modeling, or prediction within that field. If it's a specialized nomenclature, it could facilitate more precise communication and collaborative research among experts in that field. Understanding the surrounding vocabulary is critical to determining the broader implications.
- Stephanie Lai Expert Advice Insights
- Exploring Erik Estradas Net Worth How Much Is Erik Estrada Worth
To fully understand the meaning and implications of this term, more information about the specific context in which it appears is needed. This could include the associated research papers, articles, or datasets. The context will dictate the potential significance of the term within its field.
phoebekalib
Understanding the nuances of "phoebekalib" requires examining its constituent parts and context. This specialized term, likely a concept or methodology, demands careful consideration of its key elements.
- Data analysis
- Algorithm design
- Computational model
- Predictive capability
- Data structure
- Statistical method
- Scientific application
These aspects highlight the multifaceted nature of "phoebekalib." For example, its potential as a data analysis tool hinges on the sophistication of its algorithm design and its ability to produce reliable predictive models. The complexity of underlying data structures influences the suitability of statistical methods applied. Ultimately, the scientific application of "phoebekalib" depends on its efficacy and reliability within a specific domain. This complex interplay demonstrates the significant potential and critical considerations inherent in such specialized terminology.
1. Data analysis
The relationship between data analysis and "phoebekalib" is crucial but contingent on the specific meaning of "phoebekalib." Data analysis, as a fundamental process, involves examining raw data to extract meaningful insights. If "phoebekalib" represents a specific method or algorithm for data analysis, then its efficacy depends heavily on the quality and appropriateness of the underlying data analysis techniques. For instance, an algorithm designated "phoebekalib" might leverage statistical methods like regression analysis or machine learning techniques for prediction. The accuracy and reliability of "phoebekalib" directly correlate with the rigor and validity of the underlying data analysis steps.
Practical applications of such a methodology could span various fields. In healthcare, "phoebekalib" might analyze patient data to predict disease risk. In finance, it could identify patterns in market trends. In scientific research, it might aid in the interpretation of complex datasets. In each case, the efficacy of "phoebekalib" is contingent on sound data collection, appropriate preprocessing, and reliable analytical methods. If the input data is flawed or if the analytical techniques are unsuitable, "phoebekalib" will likely produce unreliable or inaccurate results.
In summary, the link between data analysis and "phoebekalib" is inseparable. The validity and usefulness of "phoebekalib" are directly tied to the robustness of the data analysis it employs. Without thorough and appropriate data analysis, "phoebekalib" cannot fulfill its intended purpose effectively. The potential applications of this methodology, therefore, depend crucially on the specific methods of data analysis that form its foundation. Careful consideration must be given to the entire data analysis pipeline to ensure the accuracy and applicability of "phoebekalib" across various domains.
2. Algorithm Design
The connection between algorithm design and "phoebekalib" is fundamental. If "phoebekalib" represents a specific methodology or approach, then the underlying algorithm dictates its capabilities and limitations. Algorithm design directly shapes the efficiency, accuracy, and applicability of "phoebekalib." A well-designed algorithm ensures "phoebekalib" functions effectively, whereas flaws in the algorithm design could lead to inaccurate results or inefficiencies. Consider, for example, a sorting algorithm central to "phoebekalib." An inefficient sorting algorithm might significantly impact the performance of the entire process. Conversely, an optimized algorithm can accelerate tasks and improve overall outcomes. In practical terms, the algorithm's ability to handle large datasets or complex input data is paramount.
The importance of algorithm design extends beyond the specifics of "phoebekalib" and is crucial in numerous data-driven applications. Modern machine learning models, for instance, rely on sophisticated algorithms for training and prediction. The design choices within these algorithms directly influence the model's accuracy and the speed of its execution. Similarly, in financial modeling, algorithmic strategies are pivotal for determining investment portfolios and risk management. The algorithm's design affects the accuracy of the predictions and the efficiency of the trading strategies. In each case, a robust algorithm forms the foundation of a successful application of "phoebekalib" and similar methodologies.
In conclusion, algorithm design is integral to "phoebekalib." The effectiveness of "phoebekalib" hinges on the efficiency and accuracy of the algorithms that drive its implementation. A carefully crafted algorithm translates to dependable outputs and enables "phoebekalib" to perform its intended tasks. Without a well-designed algorithm, "phoebekalib" may struggle to provide the expected outcomes. Understanding and appreciating the intricate relationship between algorithm design and the success of "phoebekalib" is essential for proper implementation and application within diverse fields.
3. Computational Model
A computational model, if "phoebekalib" incorporates one, is a crucial component. Such a model forms a theoretical representation of a system or process, often expressed in mathematical or algorithmic terms. The model's accuracy and fidelity directly impact the effectiveness and reliability of "phoebekalib." A robust computational model allows for the simulation and prediction of outcomes, providing a structured framework for "phoebekalib" to operate within. This is vital for any application where prediction or analysis is a key function.
Consider a scenario where "phoebekalib" is designed for forecasting financial market trends. A reliable computational model would incorporate historical data, economic indicators, and market behaviors. The model would translate these inputs into mathematical representations, allowing for the simulation of various market scenarios. The accuracy of this model is paramount. If the model inaccurately represents market dynamics, "phoebekalib" will offer flawed forecasts. Consequently, the practical significance of a well-constructed computational model becomes clear: it underpins the credibility and usefulness of the "phoebekalib" methodology. Similarly, in scientific research, accurate computational models underpin predictive simulations and aid in understanding complex phenomena. Without a suitable computational model, "phoebekalib's" predictive power is severely limited. The choice of model, its complexity, and its calibration are critical factors influencing "phoebekalib's" utility.
In essence, a computational model is foundational to "phoebekalib." Its ability to accurately represent a system or process drives the validity of any analysis or prediction undertaken by "phoebekalib." The choice of an appropriate computational model, its development, and its validation are all essential steps for constructing a reliable and insightful methodology. Robustness and accuracy are essential features of an effective computational model, and these factors directly translate to the reliability and usefulness of "phoebekalib" in diverse fields. Flaws in the model will inevitably lead to flawed conclusions in the outputs of "phoebekalib."
4. Predictive capability
The predictive capability inherent in "phoebekalib," if it possesses such a feature, is a critical aspect. Its ability to forecast future outcomes directly influences the methodology's utility and practical application. A high degree of predictive accuracy translates to greater confidence in the conclusions drawn using "phoebekalib." Conversely, poor predictive capability renders the methodology less valuable and potentially misleading. The accuracy of predictions depends on the robustness of the underlying data, the sophistication of the algorithms employed, and the validity of the computational model. Real-world examples of successful predictive modeling in fields like finance, healthcare, and climate science demonstrate the profound impact precise predictions can have. For instance, accurate disease risk prediction allows for proactive health interventions, while precise financial forecasting enables informed investment decisions.
The predictive power of "phoebekalib" can be measured by the accuracy of its forecasts, the reliability of its predictions, and the precision of the outcomes generated. Consider "phoebekalib" as a tool for predicting customer behavior. Accurate predictions allow businesses to tailor marketing strategies, optimize product development, and increase customer satisfaction. Similarly, in environmental science, accurate predictions can aid in mitigating climate change impacts. The importance of a high predictive capability in "phoebekalib" hinges on how effectively it can translate complex data into actionable insights. In evaluating "phoebekalib," the ability to consistently generate reliable predictions will be crucial for determining its value and practical applications.
In summary, the predictive capability of "phoebekalib" is pivotal to its practical application. A robust predictive capability is essential for meaningful insights and informed decision-making. The efficacy of "phoebekalib" depends heavily on its ability to accurately forecast future outcomes across various contexts. To determine the practical utility of "phoebekalib," careful evaluation of its predictive accuracy is paramount, considering various metrics and real-world case studies. Understanding the factors that influence predictive capability is essential for evaluating the methodology's strength and potential limitations.
5. Data Structure
The structure of data is fundamental to the effectiveness of any methodology, including "phoebekalib." The way data is organized, categorized, and stored directly impacts the algorithms and models that process it. A well-structured dataset enables efficient data retrieval, manipulation, and analysis. Conversely, poorly structured data can lead to inaccuracies, inefficiencies, and unreliable results in "phoebekalib" applications. The relationship between data structure and "phoebekalib" is therefore inherently critical.
- Data Format and Representation
The specific format of data, whether tabular, hierarchical, or graph-based, influences how "phoebekalib" processes it. For example, tabular data, common in spreadsheets and databases, lends itself to certain analytical techniques. Hierarchical data, often used in organizational charts or family trees, necessitates different processing methods. The appropriate data format is crucial for "phoebekalib's" successful implementation. Choosing an unsuitable format can lead to wasted computational resources and inaccurate output.
- Data Normalization and Cleaning
Normalization and cleaning procedures are integral to data structure. Inconsistent or redundant data can significantly affect the performance of "phoebekalib." Normalization ensures data integrity and consistency. Cleaning procedures address inaccuracies and missing values in the dataset, ensuring accuracy in "phoebekalib's" calculations and predictions. Clean, normalized data forms the foundation for reliable insights derived from "phoebekalib." Unprocessed or messy data diminishes the effectiveness of the methodology.
- Data Dimensionality and Complexity
The number of dimensions and the complexity of data influence the suitability of "phoebekalib" and the computational resources needed. High-dimensional data necessitates specialized techniques, potentially making "phoebekalib" computationally expensive or even impractical. The efficiency and performance of "phoebekalib" can be directly impacted by how efficiently data is handled. Understanding the data dimensionality and complexity is vital for selecting the correct methods to ensure "phoebekalib" operates effectively.
- Data Relationships and Connectivity
The relationships and connectivity within data are essential to "phoebekalib" as well. Methods used in analyzing network relationships, such as graph databases, are vital. Analyzing relationships between variables or entities, as performed by "phoebekalib," can offer insights that may be missed otherwise. The manner in which data points are interconnected or linked to one another dictates the algorithms and models suited for their analysis.
In conclusion, the data structure forms a bedrock upon which "phoebekalib" operates. The quality, format, and structure of data significantly affect the reliability and efficiency of the entire process. A clear understanding of these elements is essential for effectively utilizing "phoebekalib" to extract accurate and meaningful insights from datasets. The right data structure enables "phoebekalib" to perform optimally, whereas incorrect or inappropriate data structures limit its effectiveness.
6. Statistical method
The selection and application of statistical methods are integral to "phoebekalib." The effectiveness of "phoebekalib" hinges on the appropriateness and accuracy of the statistical techniques employed. A robust statistical approach allows for the extraction of meaningful insights from data, ensuring the reliability and validity of "phoebekalib's" conclusions. In essence, the statistical method provides the framework for interpreting the data processed by "phoebekalib."
Consider "phoebekalib" as a tool for analyzing consumer purchasing patterns. Employing statistical methods like regression analysis allows for the identification of key factors influencing purchasing decisions, such as demographics, income levels, and product features. The application of appropriate statistical tests validates the identified relationships, lending credibility to "phoebekalib's" findings. Without these statistical methods, the data analyzed by "phoebekalib" might be susceptible to spurious correlations, potentially leading to incorrect conclusions. Similarly, in scientific research, robust statistical methodologies are crucial for establishing cause-and-effect relationships and drawing accurate inferences from experimental data. The choice of statistical tests influences the reliability of findings within the context of "phoebekalib." The appropriateness of the statistical methodology directly affects the validity and application of conclusions derived from "phoebekalib."
In conclusion, the selection of suitable statistical methods is critical for "phoebekalib." These methods determine the accuracy, validity, and reliability of insights generated by the methodology. A sound statistical approach minimizes potential biases and errors, ensuring that "phoebekalib" produces reliable and meaningful results. The choice and application of statistical methods must carefully align with the objectives and characteristics of the data being analyzed, thereby enhancing the overall effectiveness of "phoebekalib." Understanding the specific statistical methods employed is fundamental to evaluating "phoebekalib's" merits and limitations in various applications.
7. Scientific application
The scientific application of "phoebekalib" hinges on its demonstrable utility in addressing specific scientific problems. This entails translating abstract concepts and methodologies into tangible, verifiable outcomes. Successful scientific applications require a rigorous process, encompassing the meticulous formulation of hypotheses, the careful design of experiments, the rigorous collection and analysis of data, and the clear communication of results. "Phoebekalib," as a potential tool or methodology, gains importance when it can contribute meaningfully to scientific advancement in a particular domain.
Practical applications of "phoebekalib" might include its use in modeling complex systems, predicting outcomes, or enhancing the understanding of natural phenomena. For instance, if "phoebekalib" is a novel approach to analyzing astronomical data, its scientific application would manifest in the improvement of cosmological models or the identification of patterns in stellar evolution. Likewise, if "phoebekalib" represents a method for simulating biological processes, its scientific application would involve verifying hypotheses about cellular mechanisms or predicting the effects of mutations. The strength of such applications lies in their demonstrable ability to advance scientific knowledge. Real-world examples where existing methods have solved scientific problems provide a benchmark for assessing the potential of "phoebekalib" in similar situations. These examples underscore the significance of rigorous testing and validation in assessing the utility of "phoebekalib."
In summary, the scientific application of "phoebekalib" is crucial for evaluating its practical value. Its potential to address scientific challenges and contribute to a deeper understanding of the natural world defines its significance. The validity of any scientific application of "phoebekalib" ultimately hinges on the robustness of the underlying methodology, the clarity and rigor of experimental design, and the clear communication of findings. The ability to demonstrably improve scientific understanding through "phoebekalib" forms the cornerstone of its utility. Without clear scientific applications, the value of "phoebekalib" remains largely theoretical.
Frequently Asked Questions about "Phoebekalib"
This section addresses common queries about "phoebekalib," a specialized term or methodology. Clear and concise answers are provided to foster understanding and facilitate informed discussion.
Question 1: What does "phoebekalib" mean?
The meaning of "phoebekalib" is context-dependent. Without specific contextual information, a precise definition cannot be given. It might represent a unique algorithm, a specific data structure, or a novel approach to a particular problem within a specialized field. Further context, such as the associated research papers, articles, or datasets, is necessary to fully understand its meaning and implications.
Question 2: What is the importance of "phoebekalib"?
The importance of "phoebekalib" depends on its specific application and context. If it represents a groundbreaking advancement in a given field, it could contribute significantly to knowledge or problem-solving. For example, in data analysis, a well-designed "phoebekalib" algorithm could lead to more efficient processing and more accurate predictions. However, without details, its specific contribution remains unclear.
Question 3: What fields might utilize "phoebekalib"?
Potential applications depend on the nature of "phoebekalib." It might be employed in fields such as data science, computational modeling, financial analysis, healthcare research, or scientific simulations, depending on the specific implementation and intended function.
Question 4: How is "phoebekalib" evaluated?
Evaluation methods vary depending on the context. To assess "phoebekalib's" effectiveness, factors such as accuracy, efficiency, scalability, and validation within the targeted field are considered. This often requires rigorous testing and comparison with existing methodologies.
Question 5: What are the potential limitations of "phoebekalib"?
Potential limitations depend on the specific design of "phoebekalib." Factors like data requirements, computational resources, or the complexity of underlying models could restrict its applicability or performance. Without a defined structure, specific limitations remain unknown.
Understanding "phoebekalib" necessitates a detailed understanding of its specific implementation and the context in which it is used. This FAQ provides a general overview but further research within a particular field is needed for specific insights.
Moving forward, the specific context and application will guide the more in-depth analysis of "phoebekalib." Further information, such as publications or datasets, are needed to proceed.
Conclusion
The exploration of "phoebekalib" reveals a complex interplay of factors influencing its potential value and application. Without precise definition or contextualization, the term remains an abstract concept. Crucial elements, including data structure, algorithm design, computational modeling, statistical methods, and predictive capability, all contribute to the overall utility of the methodology. The term's meaning and importance hinge critically on the specific application and implementation within a given field. The efficacy of "phoebekalib," therefore, hinges on demonstrable scientific utility within its domain. Evaluation demands a meticulous analysis of these interconnected components.
Further research and development within a specific context are paramount. Rigorous testing and validation within carefully designed experiments are necessary to establish the practical viability of "phoebekalib." The ability to consistently deliver reliable and meaningful results across various scientific or analytical scenarios is a vital criterion. As such, "phoebekalib," if properly defined and implemented, holds the potential to advance knowledge and problem-solving within the specified field, but until further information is available, this is merely a speculative assertion.

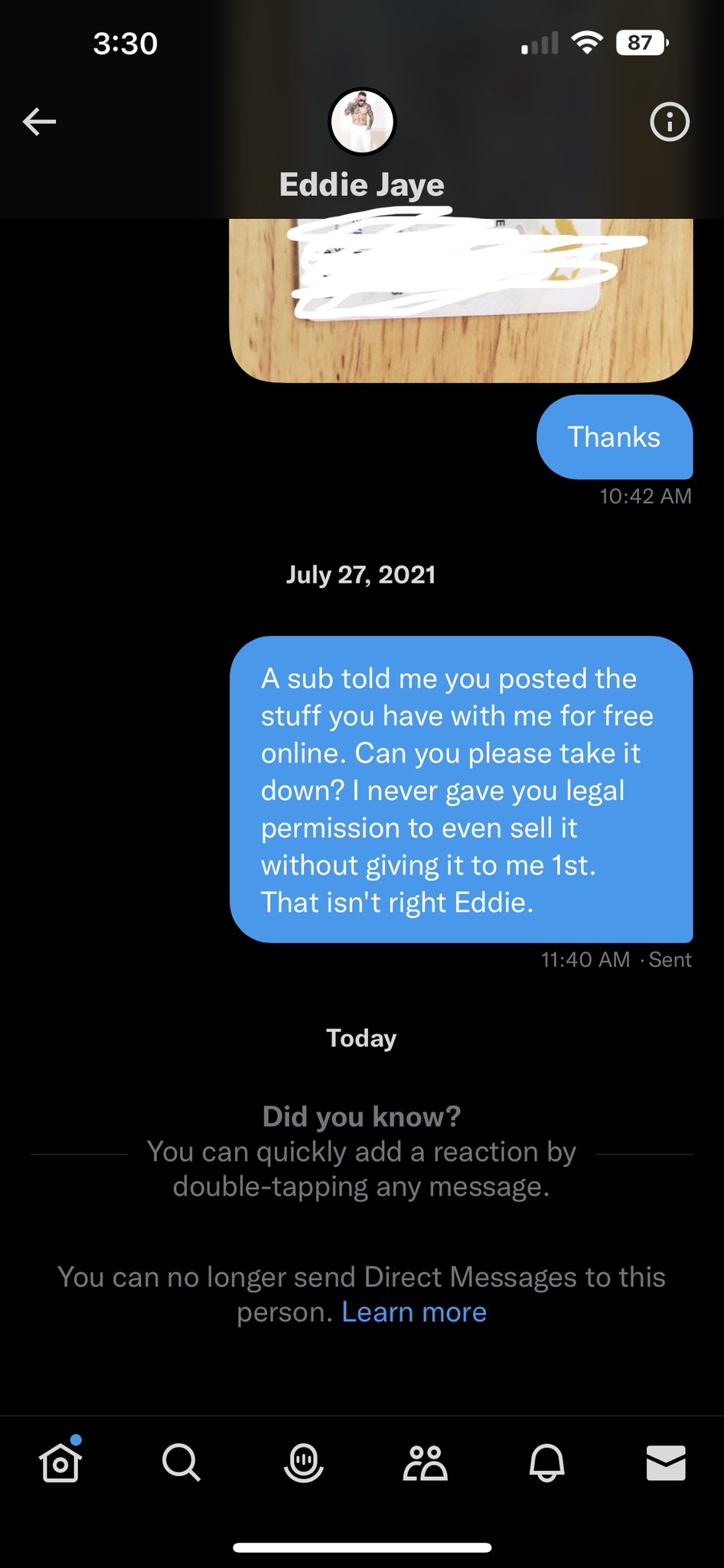
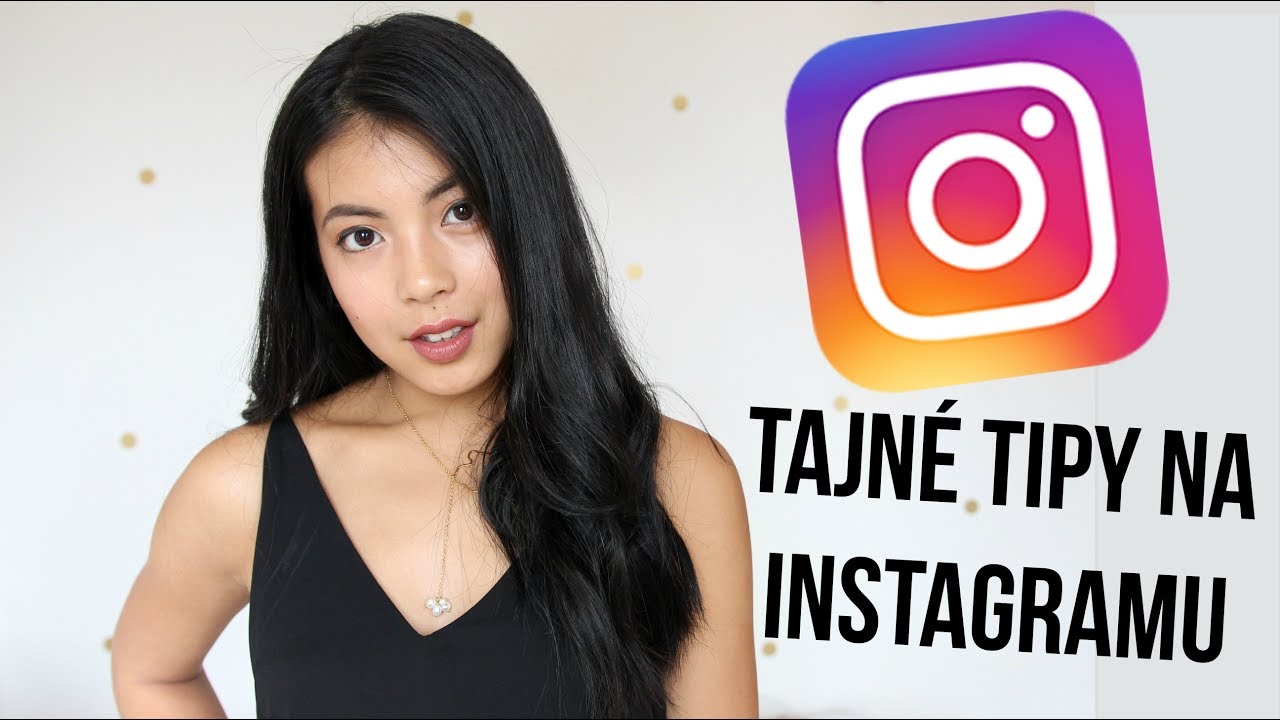
Detail Author:
- Name : Dayton Beer
- Username : wwyman
- Email : diego41@gmail.com
- Birthdate : 1988-10-03
- Address : 37850 Davis Corner Suite 707 Padbergfort, VA 28870-0857
- Phone : +1-662-370-1807
- Company : West PLC
- Job : Market Research Analyst
- Bio : Ipsam aspernatur assumenda quam deserunt rerum quidem qui. Qui inventore id non recusandae recusandae molestiae. Repellat nobis non sit blanditiis dolorem.
Socials
twitter:
- url : https://twitter.com/noah_schroeder
- username : noah_schroeder
- bio : Sint non omnis et voluptatem. Error rerum quam rerum optio voluptatum. Velit et nemo est iure. Culpa nisi harum sint accusantium sed tempora.
- followers : 161
- following : 52
facebook:
- url : https://facebook.com/schroedern
- username : schroedern
- bio : Eos quae adipisci fugiat veniam exercitationem.
- followers : 3046
- following : 614
linkedin:
- url : https://linkedin.com/in/schroedern
- username : schroedern
- bio : Eius optio qui aut tenetur.
- followers : 2246
- following : 1728
tiktok:
- url : https://tiktok.com/@noah_official
- username : noah_official
- bio : Ut itaque voluptas laborum est. Labore molestiae ut eligendi veniam dolores.
- followers : 6097
- following : 2196
instagram:
- url : https://instagram.com/noah4626
- username : noah4626
- bio : Porro ullam iure qui. Est ab cumque ad. Maiores illo cumque natus nihil vitae occaecati dolorem.
- followers : 5384
- following : 667